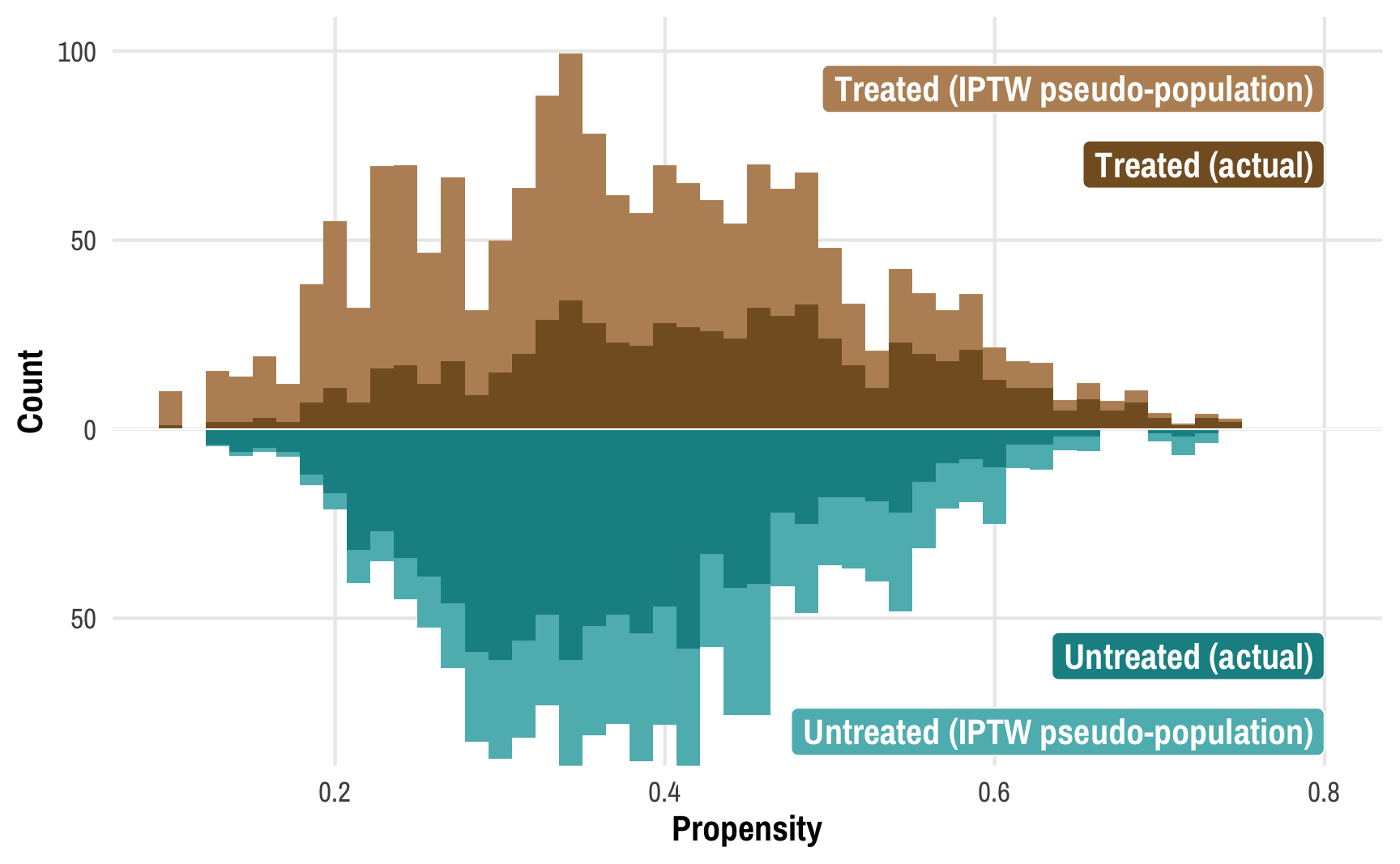
This post combines two of my long-standing interests: causal inference and Bayesian statistics. I’ve been teaching a course on program evaluation and causal inference for a couple years now and it has become one of my favorite classes ever.
This post combines two of my long-standing interests: causal inference and Bayesian statistics. I’ve been teaching a course on program evaluation and causal inference for a couple years now and it has become one of my favorite classes ever.
In most of my research, I work with country-level panel data where each row is a country in a specific year (Afghanistan in 2010, Afghanistan in 2011, and so on), also known as time-series cross-sectional (TSCS) data.
At the end of my previous post on beta and zero-inflated-beta regression, I included an example of a multilevel model that predicted the proportion of women members of parliament based on whether a country implements gender-based quotas for their legislatures, along with a few different control variables. I also included random effects for year and region in order to capture time- and geography-specific trends.
In the data I work with, it’s really common to come across data that’s measured as proportions: the percent of women in the public sector workforce, the amount of foreign aid a country receives as a percent of its GDP, the percent of religious organizations in a state’s nonprofit sector, and so on. When working with this kind of data as an outcome variable (or dependent variable) in a model, analysis gets tricky if you use standard models like
The world of econometrics has been roiled over the past couple years with a bunch of new papers showing how two-way fixed effects (TWFE; situations with nested levels of observations, like country-year, state-month, etc.) estimates of causal effects from difference-in-differences-based natural experiments can be biased when treatment is applied at different times.
Regression is the core of my statistics and program evaluation/causal inference courses. As I’ve taught different stats classes, I’ve found that one of the regression diagnostic statistics that students really glom onto is . Unlike lots of regression diagnostics like AIC, BIC, and the joint F-statistic, has a really intuitive interpretation—it’s the percent of variation in the outcome variable explained by all the explanatory variables.
A week ago we held a Community Call discussing rOpenSci Statistical Software Testing and Peer Review.This call included speakers Noam Ross, Mark Padgham, Anna Krystalli, Alex Hayes, and John Sakaluk.
Reading about someone else’s recovery-after-injury story can be a bit dull. At least that was my conclusion after pressing delete on my story a moment ago. Having spared you the details, the summary is: I got injured. It hurt. It took me a year to recover because I didn’t tackle the recovery properly.
Every week since the start of January I heard headlines like “3963 new infections, a bit more than average”. In context, that meant that the amount of positive COVID-19 that day was higher than the average daily amount of cases in the previous seven days. Even though we heard that every week, overall the cases were going down.
To validate our analyses, I’ve been using randomisation to show that the results we see would not arise due to chance. For example, the location of pixels in an image can be randomised and the analysis rerun to see if – for example – there is still colocalisation. A recent task meant randomising live cell movies in the time dimension , where two channels were being correlated with one another.