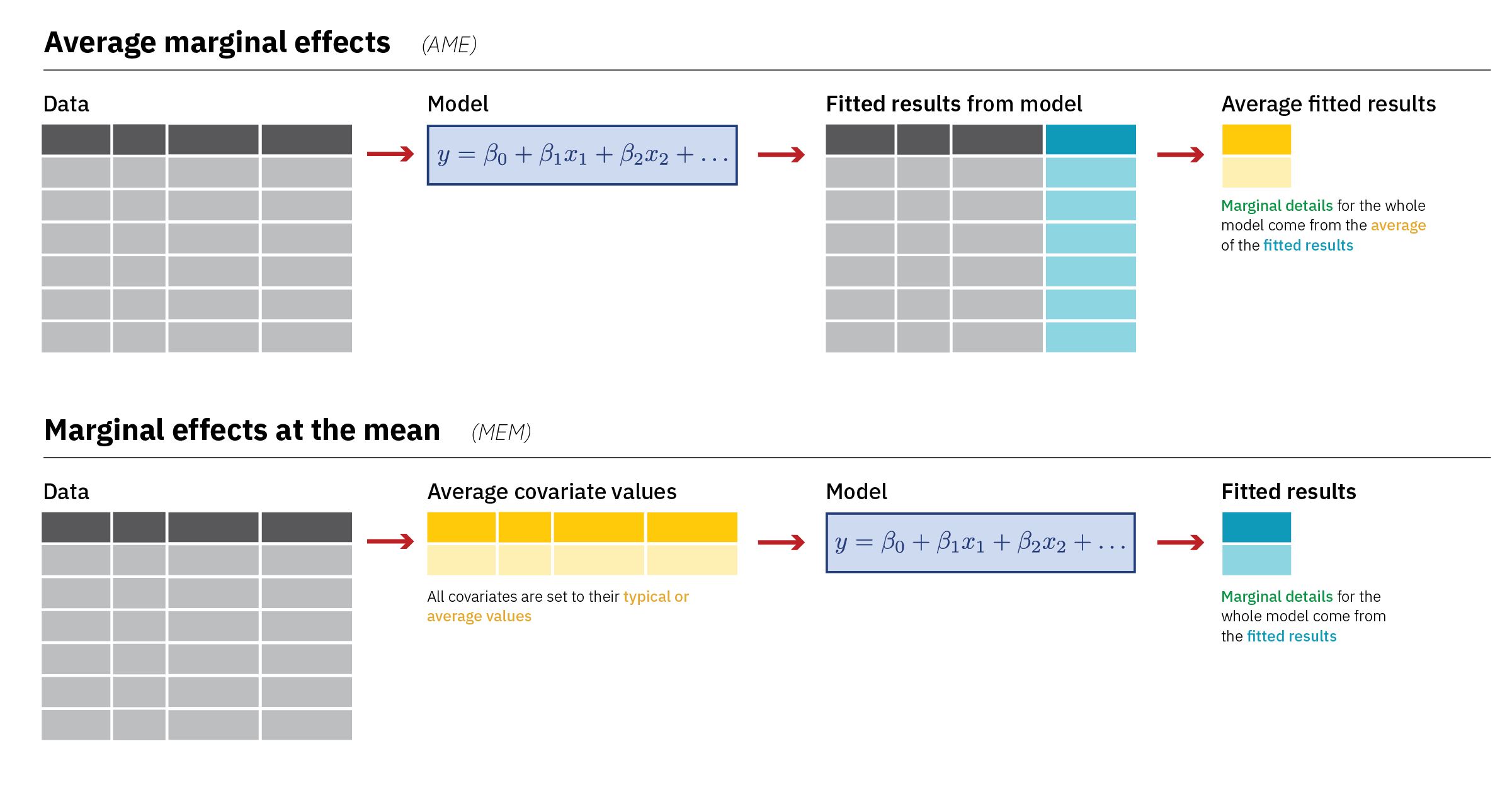
Diagrams! You can download PDF, SVG, and PNG versions of the marginal effects diagrams in this guide, as well as the original Adobe Illustrator file, here: PDFs, SVGs, and PNGs Illustrator .ai file Do whatever you want with them! They’re licensed under Creative Commons Attribution-ShareAlike (BY-SA 4.0). I’m a huge fan of doing research and analysis in public.