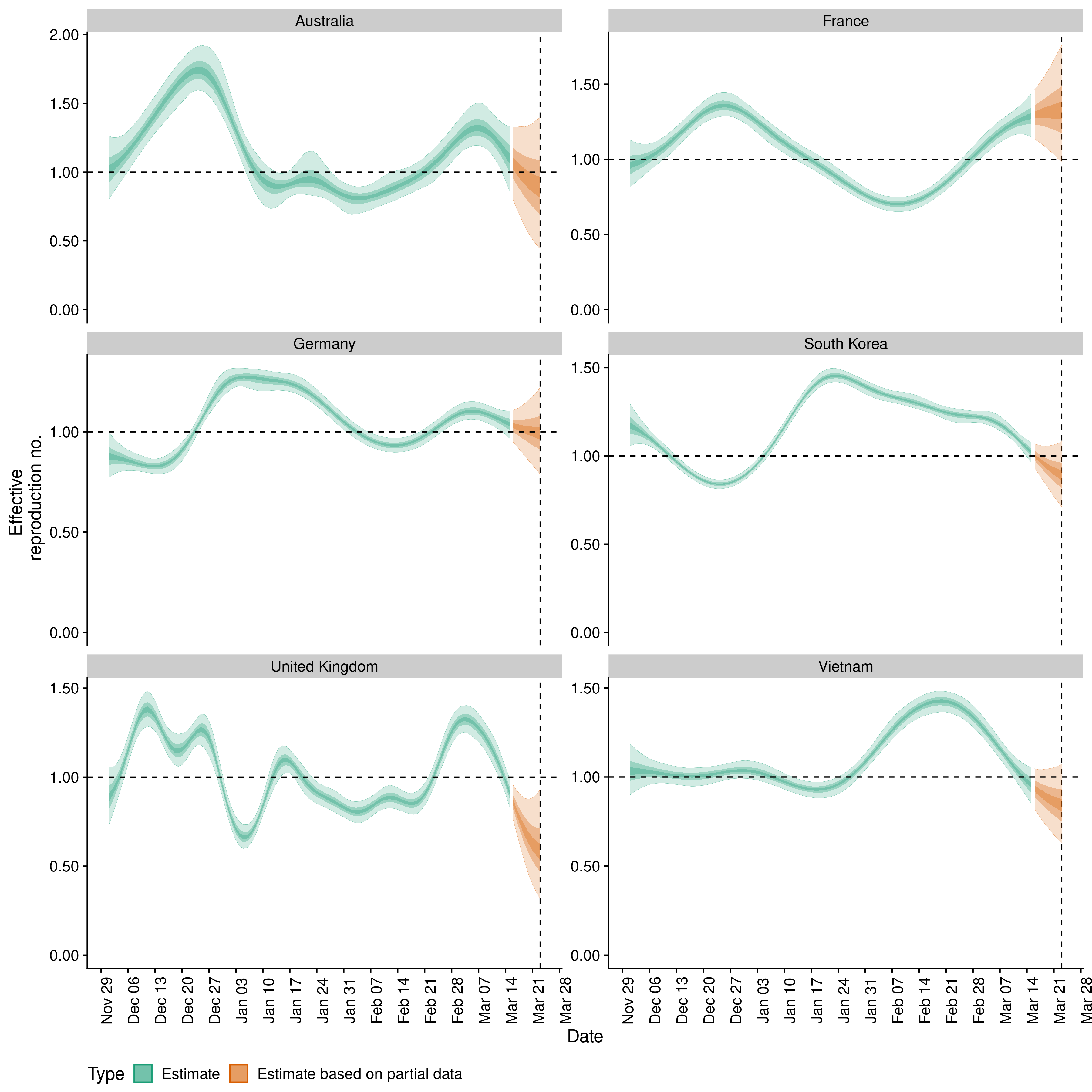
31 March, in just under a weeks time, will mark the last day we are producing global national and subnational Rt estimates and nowcasts at https://epiforecasts.io/covid/posts/global/ - more than 2 years after we published the first set of estimates. This is a good opportunity to reflect on what we have learned from this, what went well and what went wrong, and what we would aim to do better next time.