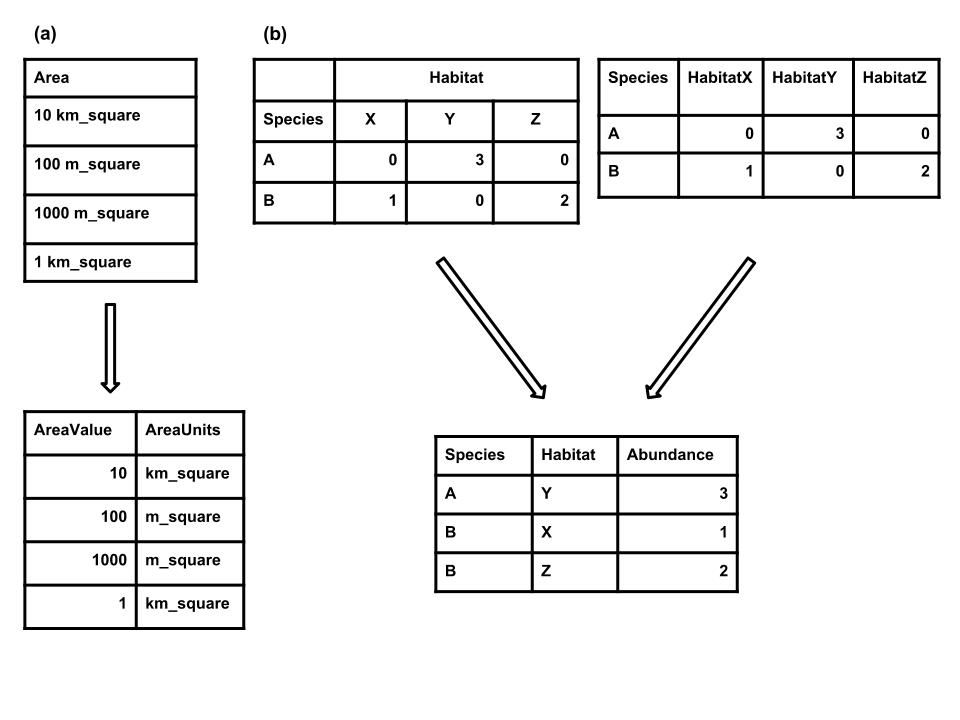
This paper in markdown format was written by Ethan White et al. The markdown file and the associated bibliogaphy and figure files are available from the Github repository of the paper. I used this version, an earlier version was published as PeerJ Preprint. Special thanks to Ethan White for allowing me to reuse this paper.