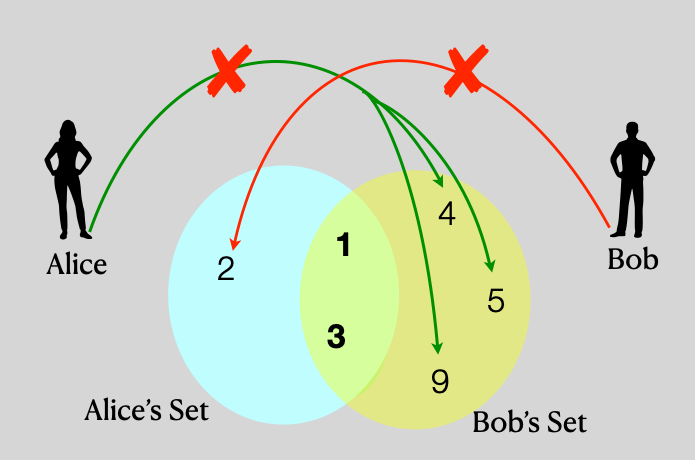
Secure multi-party computation (SMPC) enables organisations to collaborate on sensitive data analysis without directly sharing raw information. However, seemingly harmless aggregate outputs, particularly private set intersection (PSI), can leak individual-level information when analysed strategically over time.